OpenAI 今天发布几个重磅更新
1、 开放 16k 上下文的 GPT-3.5-Turbo 模型(gpt-3.5-turbo-16k),是目前的4倍 2、 新的 GPT-4 和 3.5 Turbo 模型 3、Chat Completions API 中的新增加函数调用功能 (实时获取网络数据成为可能) 4、embeddings模型的成本降低75% 5、gpt-3.5-turbo的输入token成本降低了25% (开发者的福音)
其中最令人兴奋的功能应该当属函数调用功能,我们都知道ChatGPT训练的数据是基于2021年之前的,你要问一些实时性相关的问题就没法回答你了,而函数调用让实时获取网络数据成为可能,比如查询天气预报、查股票、推荐个近期的电影之类的。
哪些模型支持函数调用?
gpt-3.5-turbo-0613
和 gpt-4-0613
这两个模型都支持函数调用。
函数调用的流程是什么?
1、用户发起提问时,调用一次带有函数描述的completions接口,gpt会判断是否支持调用函数,如果可以就从用户的提问信息中提取出函数所需要的参数。 2、开发者拿到gpt提取出来的参数后自行调用函数并返回结果 3、将函数的返回结果再次发给GPT做总结归纳为自然语言
需要注意的地方:
- 整个过程gpt会执行两次,第一次调用从问题中提取函数参数,第二次对函数返回结果做归纳总结
- 函数调用并不是由gpt调用,而是开发者来调用
举个真实例子
这里我以实时获取天气预报为例子演示函数调用的流程,天气预报接口我用的第三方高德API,免费,每天几千次的调用额度。申请注册流程这里省略,自行研究。
# 获取天气函数
def get_weather(city_name: str):
url = "https://restapi.amap.com/v3/weather/weatherInfo?"
params = {"key": "替换成你自己的key",
"city": "110000"}
city_code = "110000"
for city in CITIES:
if city_name in city.get("city"):
city_code = city.get("adcode")
break
params['city'] = city_code
response = requests.get(url=url, params=params)
# 返回结果:
# {'count': '1',
# 'info': 'OK',
# 'infocode': '10000',
# 'lives': [{'adcode': '440300',
# 'city': '深圳市',
# 'humidity': '81',
# 'humidity_float': '81.0',
# 'province': '广东',
# 'reporttime': '2023-06-14 15:00:43',
# 'temperature': '29',
# 'temperature_float': '29.0',
# 'weather': '大雨',
# 'winddirection': '西南',
# 'windpower': '≤3'}],
# 'status': '1'}
return response.json().get("lives")[0]
这个函数就是用来查询天气情况的,参数 city_name
是城市的名字,因为高德API只支持通过城市代码查天气,所以我这里做了一次根据城市名找到对应编码的查询
接口返回的结果中包含有温度(temperature)、风度(windpower)、风向(winddirection)、湿度(humidity)、天气(weather)等字段信息。
天气函数准备好后,用户开始提问:“深圳天气如何?“, 第一次调用`ChatCompletion`接口。
response = openai.ChatCompletion.create(
model="gpt-3.5-turbo-0613",
messages=[{"role": "user", "content": "深圳天气如何?"}],
functions=[
{
"name": "get_weather",
"description": "获取指定地区的当前天气情况",
"parameters": {
"type": "object",
"properties": {
"city_name": {
"type": "string",
"description": "城市,例如:深圳",
},
},
"required": ["city_name"],
},
}
],
function_call="auto",
)
这里我们指定了一个functions
参数,该参数描述了函数的名字以及参数类型,比如我们这里定义了city_name
的参数,gpt 就会从用户问题中提取出city_name。
response的返回结果是:
"choices": [
{
"finish_reason": "function_call",
"index": 0,
"message": {
"content": null,
"function_call": {
"arguments": "{\n \"city_name\": \"\u6df1\u5733\"\n}",
"name": "get_weather"
},
"role": "assistant"
}
}
],
gpt 给我们返回的message中有function_call
字段,而且 arguments 里面提取了city_name这个字段的值。
第二步:从返回结果中提取参数后调用函数, 这个过程不是交给gpt处理,而是由开发者自己调用该函数,gpt做的事情是把函数需要的参数提取出来。
message = response["choices"][0]["message"]
function_call = message.get("function_call")
if function_call:
arguments = function_call.get("arguments")
arguments = json.loads(arguments)
function_response = get_weather(
city_name=arguments.get("city_name"),
)
function_response = json.dumps(function_response)
注意,这里要将函数调用返回的结果做一些json转换
第三交步:把返回结果给gpt做总结归纳
second_response = openai.ChatCompletion.create(
model="gpt-3.5-turbo-0613",
messages=[
{"role": "user", "content": "深圳今天的天气?"},
message,
{
"role": "function",
"name": "get_weather",
"content": function_response,
},
],
)
return second_response
注意messages列表中最后一条消息中role
角色是 function, 最后得到的结果second_response
中的content内容为:
content: 深圳今天的天气是大雨,气温为25℃,风向是西北风,风力为3级,湿度为95%。
最后,我们把逻辑集成到我们微信中得到的效果是这样的:
完整源代码:https://gist.github.com/lzjun567/04310ae22dc80005997179c1e591b397
关注公众号「Python之禅」,回复「1024」免费获取Python资源
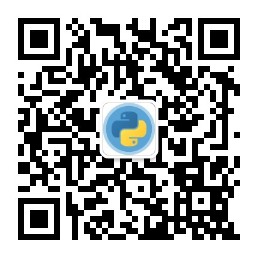